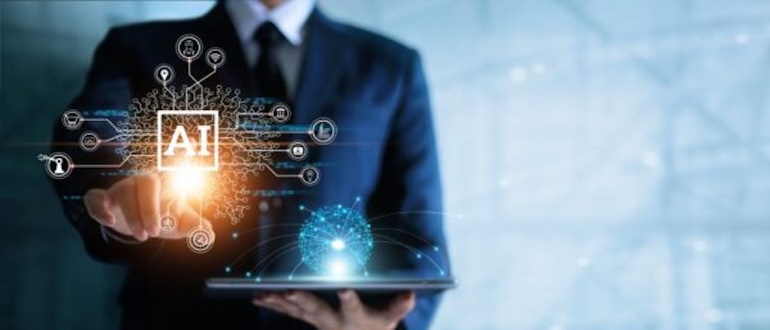
NTT DATA has added a portfolio of consulting and managed services to help organizations build and deploy artificial intelligence (AI) agents.
The breadth of NTT DATA Agentic AI Services for Hyperscaler AI Technologies offering spans everything from providing advice on how to best build and apply AI agents to managing them on the behalf of companies, says Charlie Doubek, global vice president for managed collaboration and communications at NTT DATA.
Initially focused on Microsoft cloud platforms, the services leverage a set of low-code tools NTT Data has built to enable organizations to design and build AI agents. A recent NTT Data survey found that 96% of the CIOs and CTOs surveyed prefer a cloud-based approach to agentic AI.
NTT Data earlier this year launched its own Smart AI Agent capable of automating a wide range of tasks. That offering includes retrieval augmented generation (RAG) technologies to expose large language models (LLMs) to additional data along with a framework that enables multiple AI agents to collaborate. At the time of the launch of Smart AI Agent, NTT Data predicted these technologies would drive an additional $2 billion in revenue by 2027.
It’s not clear how quickly AI agents will be deployed but the bulk of existing business processes in the months and years ahead will be re-engineered to accommodate them. Many of the rote tasks that today are performed by employees will increasingly be offloaded to an AI agent that has been specifically trained using the reasoning capabilities exposed by an LLM to automate specific tasks.
The challenge then becomes how to orchestrate the management of an end-to-end task being performed by AI agents that are supervised by human managers.
It may be a while yet before AI agents are routinely performing complex tasks, but it’s already apparent many simpler tasks can be automated using an AI agent. The issue is that AI agents are based on LLMs that are probabilistic in the sense they are providing output that represents a best guess. Most of the time that output is accurate but some business processes are deterministic in the sense they need to be performed the same way every time. AI agents will be increasingly trained using narrow sets of data to limit the scope of the outputs that might be generated, but it remains advisable to ensure those outputs are reviewed before being automatically implemented.
In fact, most organizations as such would be well advised to focus on relatively simple use cases to ensure there is an actual return on investment (ROI), says Doubek. “You need to look at the use case,” he says.
Regardless of whether organizations rely on external partners or opt to do most of the work themselves, the level of business process re-engineering that is about to occur will surpass any previous era as AI agents move from being seen as a technology that provides a competitive advantage to the new table stakes for remaining competitive. The issue, of course, is determining how quickly to implement those AI agents based on not just their ability to execute a task but also over the long term total cost of invoking them.