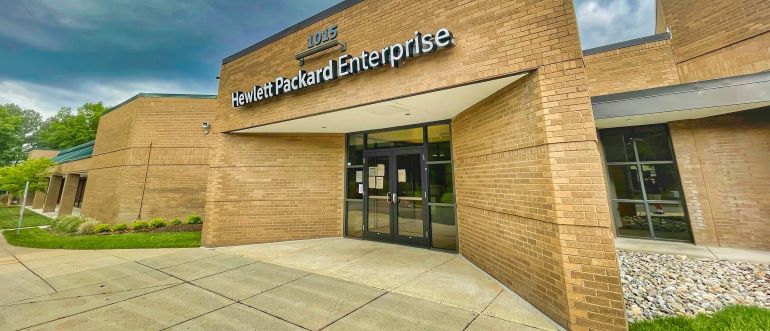
Hewlett Packard Enterprise (HPE) today added additional platforms to its portfolio for deploying artificial intelligence (AI) applications using the latest generation of graphics processing units (GPUs) from NVIDIA.
Additionally, HPE announced that its data storage and networking technologies will be integrated with similar technologies that NVIDIA is using to create the NVIDIA AI Data platform for accessing customizable blueprints for building AI applications.
Announced at the NVIDIA GTC AI 2025 conference, the goal is to bring the NVIDIA Data Platform to the HPE GreenLake service through which the company both manages IT infrastructure on behalf of its customers and provides access to a data lakehouse that can be used for training AI models.
HPE is also updating its OpsRamp platform for applying AI to IT operations to include an ability to optimize consumption of GPU resources.
Finally, the company is also launching a Private Cloud AI developer system to provide access to tooling and 32TB of integrated storage.
Collectively, these capabilities will dramatically reduce the amount of time needed to build out a data center for running AI applications, says Fidelma Russo, executive vice president and general manager for Hybrid Cloud, and CTO at HPE. “AI data centers can now be created in months rather than years,” she adds.
For example, HPE has created AI Mod POD, a 1.5 MW module system that makes use of liquid cooling to enable organizations to deploy AI workloads in a way that reduces energy requirements.
It’s not clear to what degree IT infrastructure is holding back the building and deployment of AI applications. While organizations can build and deploy AI applications in the cloud, many of them need to be deployed closer to the point where data is created and stored in on-premises IT environments.
Many organizations, because of compliance requirements or security concerns, may also simply prefer to both train and deploy AI models in on-premises IT environments.
Regardless of motivation, the HPE GreenLake service promises to provide access to GPUs for AI applications in a way that doesn’t necessarily require organizations to acquire and retain their own internal IT expertise, notes Russo.
That may prove critical at a time when the cost of building applications infused with AI capabilities is increasing.
Organizations are also clearly anxious to build and deploy AI applications as quickly as possible but may not be entirely sure of how a return on that investment might be achieved. Relying more on a managed service should provide those organizations with greater flexibility when it comes to allocating IT infrastructure resources to specific initiatives.
Ultimately, each organization will need to determine what class of processors makes the most sense for running any given workload at any given time. The challenge, as always, is the class of servers being successfully used to run an AI workload today might not be fit for that purpose a few short months later. In fact, outsourcing the management of IT infrastructure at a time when little is known about how AI workloads will behave in a production environment might even prove to be the only prudent choice available.