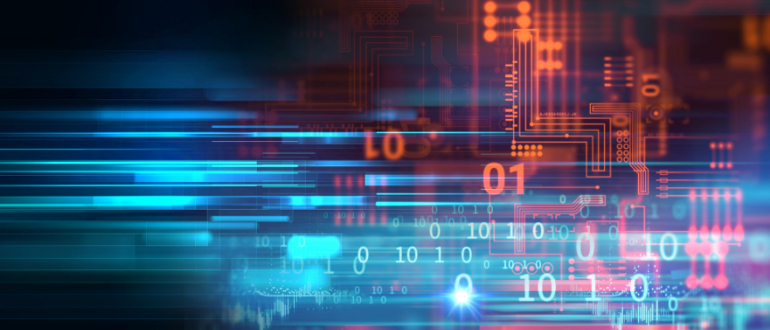
The other day, my son was grabbing his sister’s phone to bring it to her. He was shocked when he glanced at the screen and the phone unlocked. He knew there was no way his sister would have given him access. So why did his face open the device?
The reason is my kids are mixed race. The algorithm couldn’t tell their faces apart. You can imagine their dismay when my kids realized that they didn’t have the security or privacy they thought was built into the device for all.
Sadly, this situation is not unique. My car too fails to recognize my slightly accented voice commands. Many others face similarly frustrating issues. Artificial intelligence (AI) is all too often not fed diverse data, and those that lay outside the majority find themselves exposed to security issues or inaccessibility to key features.
Why do these keep happening, and how can we prevent them?
Seeking diversity isn’t just lip service done to appear modern and inclusive. Committing to using representative data and inputs help our products and services reach every demographic without embarrassing — and unacceptable — mishaps.
You Only Have One Shot
AI innovations are flooding the marketplace, bringing exciting and impactful technologies to the users and enterprises. But with an increasingly crowded market, many companies only have one chance at making a good impression. A single failure, such as an AI being unable to recognize a certain ethnicity or showing a gender bias, can tarnish the company’s name forever. People are already skeptical of AI and they only need one more reason to push a provider to oblivion. Failing to put a proper emphasis on inclusive data can ensure this easy death.
Starting from the Bottom
As we know, getting the right data to feed an AI system is of utmost importance. And from the very first moments of conception, engineers need to intentionally implement data that is not only high quality but also richly diverse into training. These are some key traits to look out for:
- Linguistic Diversity: As I encountered with my car’s voice command system, a lack of linguistic diversity can shut out many users from accessing useful features in an applications. When building a voice-driven AI, consider training on not only different languages but also a wide range of sounds and accents, so that the AI is better prepared to understand every variation of a given language.
- Visual Diversity: Tagging issues often arise from a lack of attention to visual diversity. In one case, AI labeled black men as “primates” in photos. Exposing AI to a wide range of people of every race, background, gender and age can eliminate such preposterous biases and produce accurate and helpful results.
- Content Diversity: I would hate to see an AI that was only trained on Reddit posts. AI should be trained on content from a variety of sources, including books, websites, social media and peer-reviewed papers. This will obviously vary depending on the AI itself, but a better range of content will help it understand the nuances of situations, avoiding embarrassing misunderstandings.
These are only a few of the basic elements to consider, but of course, methods vary and one can go as deep as they need to depending on the type of AI they are building.
One may argue that collecting the right set of data from get go can cost extra resources and make the project cost-heavy, which can no doubt be frustrating in the quick-moving tech world, but while speed is valuable, mistakes are fatal, and in business its important to make that distinction early.
Keeping It up
So we’re off to a great start, but how do we ensure that diversity is continuously a point of focus?
For a topic that’s ever-evolving, proactivity is key. This can be a relatively easy task in certain areas, such as when the AI is tied to a product like hardware or wearables. The product naturally needs updates and new releases, and any design change will naturally impact the algorithm. With these updates, changes and refreshes, the diversity aspect of the AI can be easily included.
Other AI systems might require more intentional refreshes, and the updates should be built into a regular schedule to ensure they are not overlooked.
Meeting a New Need
The idea that AI is stealing jobs is widespread, and many fear being replaced by AI tools and technologies. Putting emphasis on inclusion is a way of telling the workforce that contrarily more AI-related jobs can be created.
As AI programs become more human-focused with augmented reality, wearables and AI assistants, inclusive AI will only become more vital. Data professionals who specialize in this area are becoming increasingly valuable. Moreover, human experts are necessary for bias mitigation and solving problems on-the-go. Training employees to specialize in this area will reap long-term rewards for both individuals and the team.
Getting Ahead of the Curve
Regulation is often far behind innovations, and that is also the case with AI. As of now, there are no training or data standards for AI, which has only helped exacerbate these issues. But many, myself included, are rallying for change, which is on the horizon. Those who fail to implement diverse training will fall behind when regulations hit, and those who have implemented these standards from the beginning will find themselves bounding ahead.
In an increasingly flooded marketplace, we can differentiate ourselves from the rest by committing to diverse AI. I, for one, would like to see a world where my kids, and those outside the so-called “norm” can access every AI feature and benefit from its valuable functions like everybody else. AI has incredible potential, let’s not leave anyone behind.