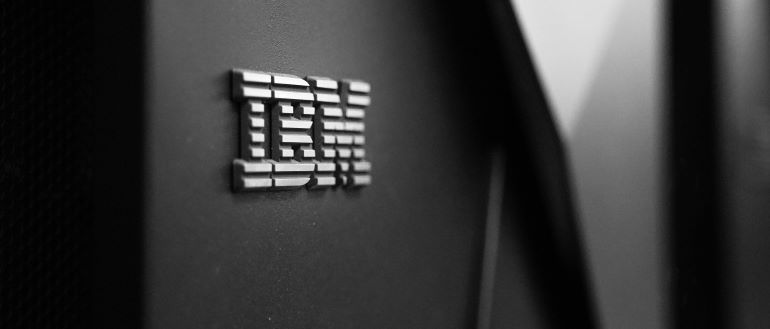
IBM has launched its most advanced family of AI models, Granite 3.0, built on 12 trillion tokens from 12 natural languages and 116 programming languages.
The models are designed for enterprise AI needs with performance in tasks such as classification, summarization and retrieval augmented generation (RAG).
With a focus on transparency and safety, IBM is also offering technical report detailing datasets, filtering processes and performance benchmarks.
The models, available under the Apache 2.0 license, offer flexibility for businesses to fine-tune them with proprietary data and integrate the InstructLab alignment technique, introduced in collaboration with RedHat.
Additionally, IBM provides IP indemnity for all Granite models on watsonx.ai, to boost confidence for enterprise clients merging their proprietary data with the models.
The Granite 3.0 family includes a general purpose/language set, Guardrails & Safety and the “Mixture-of-Experts” (MoE) models, such as the 1B-A400M and 3B-A800M, designed for low-latency and CPU-based deployments.
The unique RAG checks, designed to help ensure accuracy in context and answer relevance, can be integrated with any open or proprietary AI models.
Granite Instruct, Guardian Variants
The Granite models come in two variants: Instruct and Guardian. The Instruct models are designed for general-purpose tasks such as language processing, coding and multilingual applications, functioning like typical large language models.
The Guardian models are specialized companions that enhance security by detecting potential harms or adversarial attacks, both on model inputs and outputs.
These models are fine-tuned versions of the original Granite models, designed to identify risks like hallucinations and biased content.
Guardian models also provide risk detection, allowing developers to implement guardrails by checking user prompts and AI responses for issues like social bias, toxicity and profanity.
Kate Soule, program director for IBM’s data and model factory, said the company plans to expand the Guardian models’ capabilities, including improved hallucination detection for workflows involving tool use and external API calls.
“The Guardian models are not limited to the Granite ecosystem—they can be deployed alongside any AI model,” she said. “We believe in providing a diverse toolkit of models for enterprises to choose from.”
Building Responsible AI
Soule added IBM is committed to responsible AI, noting transparency is at the core of that commitment.
She explained the Granite models are rated highly by Stanford’s transparency index, a recognition IBM aims to uphold with its most recent release.
“We have published a detailed technical paper and responsible use guide, outlining every dataset used to train the models and how that data was curated,” Soule said.
This open approach is designed to encourage both the open source community and enterprise customers to build on and customize the models for their own use cases.
“We want users to understand what these models have been trained on so they can confidently bring their own data into the process and fine-tune them further,” she explained.
From Soule’s perspective, there is significant value for enterprises in building on top of GenAI models by integrating proprietary data, which is often the cornerstone of a business.
“We want people to build on these models, especially by bringing their enterprise’s prize IP into the process and fine-tuning the models with that data,” she said.
Ensuring that companies retain full control over their intellectual property was a key factor in releasing the Granite models under the Apache 2.0 license.
“It was really important to release these models under terms that give enterprise customers confidence that they have full rights to how their IP is used in the model,” she explained.
This approach ensures companies can leverage tools like Red Hat’s InstructLab without worrying about restrictions on their IP.
Cost-Effective AI With Guardrails
Soule said businesses need more than just AI tools to make their investments meaningful—they must focus on cost-efficiency, safety and speed of deployment.
“You can’t automate something if it’s more expensive to run a 400-billion-parameter model than to do the job manually,” she said. “Ensuring AI models are cost-effective and equipped with proper guardrails is critical.”
She explained the current AI landscape is shifting towards smaller, powerful models that can handle real-world tasks like RAG customer support, and content creation.
While IBM plans to release larger models in 2025, Soule said the focus remains on providing efficient, smaller models capable of powering today’s AI use cases effectively.
“IBM believes the workhorse size for LLMs will be under 20 billion parameters, handling 90% of production tasks,” Soule said.