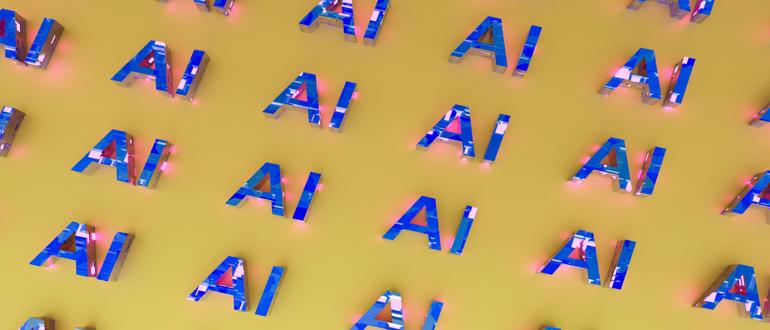
Generative artificial intelligence- (AI) powered technologies have become an everyday part of our lives, whether they’re summarizing our emails, conducting image-to-image translation or providing helpful answers via a chatbot. The advancements we’ve seen thus far are only the tip of the iceberg, but as with any disruptive technology, companies will need to adjust their strategy to realize AI’s full potential.
Given this, it’s no surprise AI has significantly altered the way organizations think about data, cloud migration and privacy. Let’s explore AI’s impact on each of these areas, including how enterprises can mitigate new challenges or security gaps that may arise.
Data: Once You Share It, There’s No Going Back
On their own, foundation models created in the public cloud using open source data are only minimally (if at all) useful to an enterprise. Where the magic happens is when companies train these models with their own proprietary data. For example, a company might use a foundational model trained on its HR data to create a chatbot that lets employees ask questions about their benefits, corporate policies and more.
As the name suggests, these models serve as the literal foundation for generative AI tools and applications. But organizations need to exercise an abundance of caution when training them, because once they have access to company data, there’s no going back. Think of it like spilling a secret: As soon as you divulge private information to someone, they can’t unhear or “unknow” it.
In addition to training foundational models, enterprises must also conduct inferencing to verify that their models are making accurate predictions and decisions. Inferencing must occur within the four walls of the enterprise—on specific tool chains—in order to protect proprietary data and prevent it from leaking back into the foundational model. Notably, inferencing is notoriously energy-intensive, so enterprises also need to make their models as compact as possible (without sacrificing security) in order to keep power consumption in check.
Cloud Migration: AI Is the Ultimate Hybrid Cloud Application
The processes outlined above illustrate why AI-based services and applications are made for hybrid cloud and multi-cloud architectures. While the public cloud will fuel a significant portion of AI development (public cloud services are forecast to reach a whopping $1 trillion by 2027), enterprises still want the ability to manage their proprietary data in private clouds or their own data centers. A hybrid cloud or multi-cloud IT model gives them the ability to freely move their AI workloads between various infrastructure environments.
Here’s what that looks like: Training takes place in the public cloud; refinement and retrieval-augmented generation (RAG) takes place on-premises (typically in core data centers), and inferencing should generally occur at the edge in order to save on the aforementioned power consumption. Occasionally, inferencing will occur in the public cloud and enterprises can provide an inferencing endpoint for client applications. But any way you slice it, there’s no way to fully embrace the power of AI without the support of a hybrid cloud architecture.
Privacy: You Can’t Be Too Careful
Since AI systems often contain sensitive data, privacy concerns can emerge. First, there’s a risk of proprietary data leaking back into the foundation model. This could result in a company’s “secret sauce” becoming public information. Second, there’s a chance of sensitive company data being exposed through a security breach. Cybercriminals know AI systems house sensitive data, making them a prime target.
Additionally, unauthorized access can become an issue if the parameters for AI algorithms aren’t clearly defined. Think back to the HR chatbot example previously referenced; imagine an entry-level employee gaining access to HR data (like payroll information, for example) that should only be accessible to executives. Finally, if an organization’s training data isn’t diverse enough, users may be able to zero in on specific individuals through the process of elimination. Many of these concerns can be mitigated or eliminated by proper data cleansing and processing. Additionally, data encryption and multicollinearity detection to ensure data isn’t too strongly correlated can further enhance data privacy.
AI has unlocked a plethora of exciting opportunities for companies to innovate—and this is just the start. Consequently, organizations need to think about data, cloud migration and privacy in new ways in order to succeed in the age of AI. By exercising caution during model training, embracing a hybrid cloud or multi-cloud architecture and strengthening data privacy, enterprises can be well on their way to making AI-driven breakthroughs, safely and efficiently.